The Internet of Things (IoT) solutions require data integration capabilities to help business leaders solve real problems. Ecosystm research finds that the problem is that more than half of all organisations are finding integration a key challenge – right behind security (Figure 1). So, chances are, you are facing similar challenges.
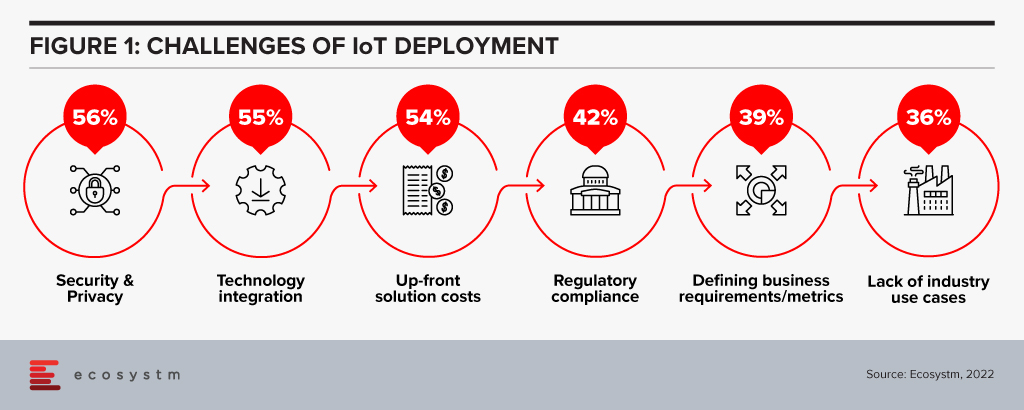
This should not be taken as a criticism of IoT; just a wake-up call for all those seeking to implement what has long been test-lab technology into an enterprise environment. I love absolutely everything about IoT. IT is an essential technology. Contemporary sensor technologies are at the core of everything. It’s just that there are a lot of organisations not doing it right.
Like many technologists, I was hooked on IoT since I first sat in a Las Vegas AWS re: invent conference breakout session in 2015 and learned about MQTT protocols applied to any little thing, and how I could re-order laundry detergent or beer with an AWS button, that clumsy precursor to Alexa.
Parts of that presentation have stayed with me to this day. Predict and act. What business doesn’t want to be able to do that better? I can still see the room. I still have those notes. And I’m still working to help others embrace the full potential of this must-have enterprise capability.
There is no doubt that IoT is the Cinderella of smart cities. Even digital twinning. Without it, there is no story. It is critical to contemporary organisations because of the real-time decision-making data it can provide into significant (Industry 4.0) infrastructure and service investments. That’s worth repeating. It is critical to supporting large scale capital investments and anyone who has been in IT for any length of time knows that vindicating the need for new IT investments to capital holders is the most elusive of business demands.
But it is also a bottom-up technology that requires a top-down business case – a challenge also faced by around 40% of organisations in the Ecosystm study – and a number of other architectural components to realise its full cost-benefit or capital growth potential. Let’s not quibble, IoT is fundamental to both operational and strategic data insights, but it is not the full story.
If IoT is the belle of the smart cities ball, then integration is the glass slipper that ties the whole story together. After four years as head of technology for a capital city deeply committed to the Smart City vision, if there was one area of IoT investment I was constantly wishing I had more of, it was integration. We were drowning in data but starved of the skills and technology to deliver true strategic insights outside of single-function domains.
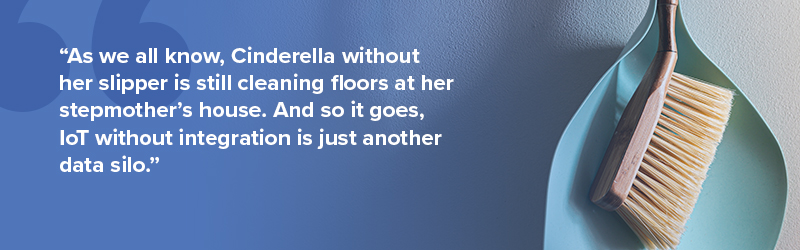
This reality in no way diminishes the value of IoT. Nor is it either a binary or chicken-and-egg question of whether to invest in IoT or integration. In fact, the symbiotic market potential for both IoT and integration solutions in asset-intensive businesses is not only huge but necessary.
IoT solutions are fundamental contemporary technologies that provide the opportunity for many businesses to do well in areas they would otherwise continue to do very poorly. They provide a foundation for digital enablement and a critical gateway to analytics for real-time and predictive decision making.
When applied strategically and at scale, IoT provides a magical technology capability. But the bottom line is that even magic technology can never carry the day when left to do the work of other solutions. If you have already plunged into IoT then chances are it has already become your next data silo. The question is now, what you are going to do about it?
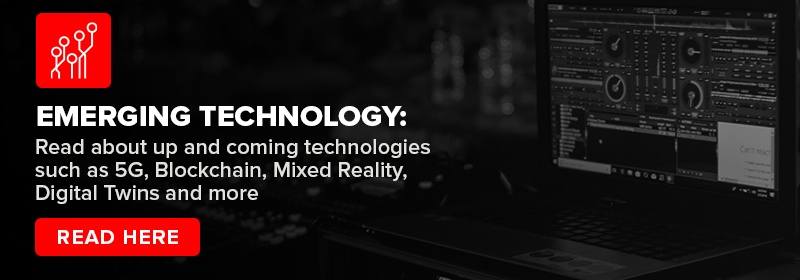
Cities worldwide have been facing unexpected challenges since 2020 – and 2022 will see them continue to struggle with the after-effects of COVID-19. However, there is one thing that governments have learnt during this ongoing crisis – technology is not the only aspect of a Cities of the Future initiative. Besides technology, Cities of the Future will start revisiting organisational and institutional structures, prioritise goals, and design and deploy an architecture with data as its foundation.
Cities of the Future will focus on being:
- Safe. Driven by the ongoing healthcare crisis
- Secure. Driven by the multiple cyber attacks on critical infrastructure
- Sustainable. Driven by citizen consciousness and global efforts such as the COP26
- Smart. Driven by the need to be agile to face future uncertainties
Read on to find out what Ecosystm Advisors, Peter Carr, Randeep Sudan, Sash Mukherjee and Tim Sheedy think will be the leading Cities of the Future trends for 2022.
Click here to download Ecosystm Predicts: The Top 5 Trends for Cities of the Future in 2022
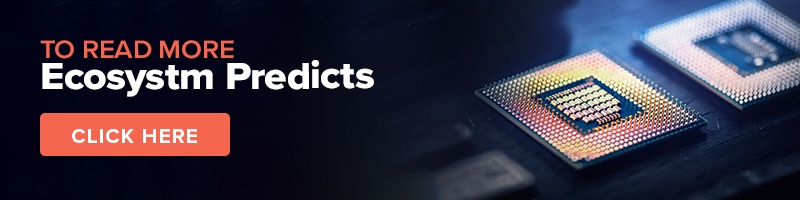
One of the biggest impacts of the pandemic has been the uptick in cloud adoption. Ecosystm research shows that more than half the organisations are either building cloud native applications or have a Cloud-First strategy. Cloud infrastructure, platforms and software became a key enabler of the business agility and innovation that organisations needed to survive and succeed.
However, as organisations look to become data-driven and digital, they will require seamless access to their data, irrespective of where they are generated (enterprise systems, IoT devices or AI solutions) and where they are stored (public cloud, Edge, on-premises or data centres) to unlock the full value of the data and deliver the insights needed. This will shape the Cloud and Data Centre ecosystem in 2022.
Read on to find out what Ecosystm Analysts, Claus Mortensen, Darian Bird, Peter Carr and Tim Sheedy think will be the leading Cloud & Data Centre trends in 2022.
Click here to download Ecosystm Predicts: The Top 5 Trends for Cloud & Data Centre in 2022 as PDF
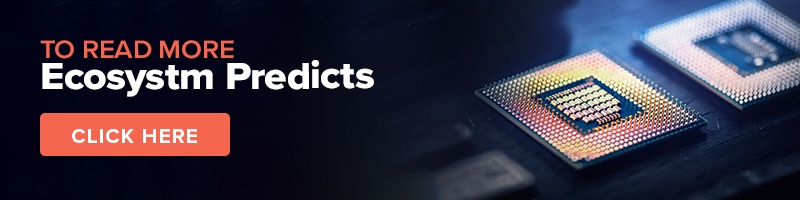
In this Insight, guest author Anupam Verma talks about how a smart combination of technologies such as IoT, edge computing and AI/machine learning can be a game changer for the Financial Services industry. “With the rise in the number of IoT devices and increasing financial access, edge computing will find its place in the sun and complement (and not compete) with cloud computing.”
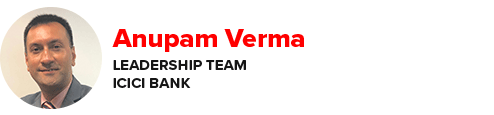
The number of IoT devices have now crossed the population of planet earth. The buzz around the Internet of Things (IoT) refuses to go down and many believe that with 5G rollouts and edge computing, the adoption will rise exponentially in the next 5 years.
The IoT is described as the network of physical objects (“things”) embedded with sensors and software to connect and exchange data with other devices over the internet. Edge computing allows IoT devices to process data near the source of generation and consumption. This could be in the device itself (e.g. sensors), or close to the device in a small data centre. Typically, edge computing is advantageous for mission-critical applications which require near real-time decision making and low latency. Other benefits include improved data security by avoiding the risk of interception of data in transfer channels, less network traffic and lower cost. Edge computing provides an alternative to sending data to a centralised cloud.
In the 5G era, a smart combination of technologies such as IoT, edge computing and AI/machine learning will be a game changer. Multiple uses cases from self-driving vehicles to remote monitoring and maintenance of machinery are being discussed. How do we see IoT and the Edge transforming Financial Services?
Before we go into how these technologies can transforming the industry, let us look at current levels of perception and adoption (Figure 1).
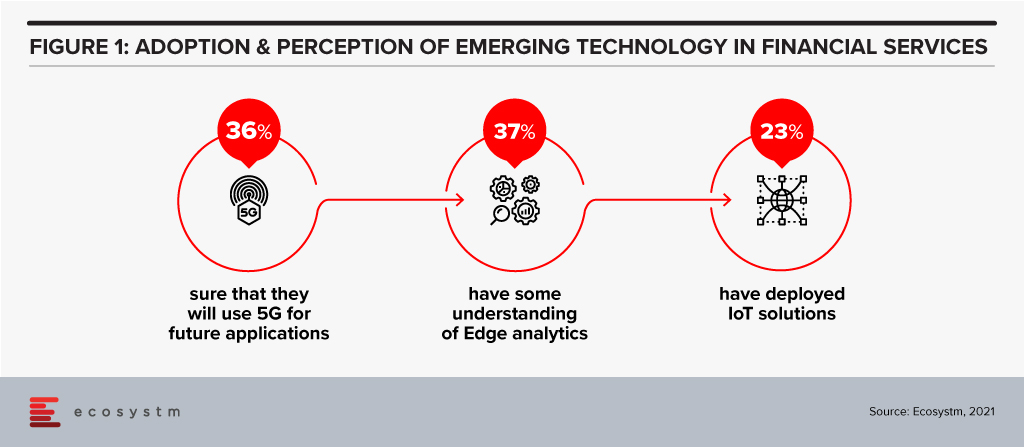
There is definitely a need for greater awareness of the capabilities and limitations of these emerging technologies in the Financial Services.
Transformation of Financial Services
The BFSI sector is increasingly moving away from selling a product to creating a seamless customer journey. Financial transactions, whether it is payment, transfer of money, or a loan can be invisible, and Edge computing will augment the customer experience. This cannot be achieved without having real-time data and analytics to create an updated 360-degree profile of the customer at all times. This data could come from multiple IoT devices, channels and partners that can interface and interact with the customer. A lot of use cases around personalisation would not be possible without edge computing. The Edge here would mean faster processing and smoother experience leading to customer delight and a higher trust quotient.
With IoT, customers can bank anywhere anytime using connected devices like wearables (smartwatches, fitness trackers etc). People can access account details, contextual offers at their current location or make payments without even needing a smartphone.

Use Cases of IoT & Edge in Financial Services
IT and Digital Leaders in Financial Services are aware of the benefits of IoT and there are some use cases that most of them think will help transform Financial Services (Figure 2).
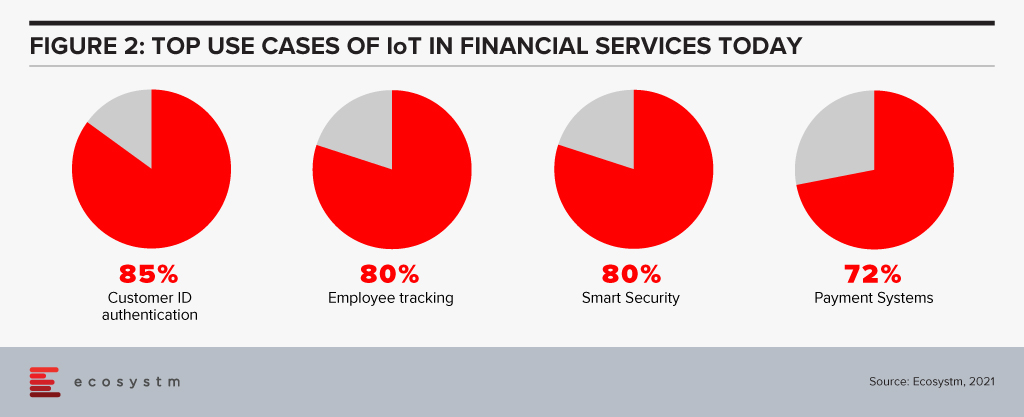
However, there are many more potential use cases. Here are some use cases whose volume will only grow every day to fuel incessant data generation, consumption and processing at the Edge.
- Smart Homes. IoT devices like Alexa/Google Home have capabilities to become “bank in a speaker” with edge computing.
- In-Sync Omnichannels. IoT devices can be synced with other banking channels. A customer may start a transaction on an IoT device and complete it in a branch. Facial recognition can be used to identify the customer after he/she walks in and synced IoT devices will ensure that the transaction is completed without any steps repeated (zero re-work) thereby enhancing customer satisfaction.
- Virtual Relationship Managers. In a digital branch, the customer may use Virtual Reality (VR) headsets to engage with virtual relationship managers and relevant experts. Gamification using VR can be amazingly effective in the area of financial literacy and financial planning.
- Home and Auto Purchase. VR may also find use in home and auto purchase processes with financing built into it. The entire customer journey will have a much smoother experience with edge computing.
- Auto and Health Insurance. Companies can use IoT (device installed in the vehicle) plus edge computing to monitor and improve driving behaviour, eventually rewarding safety with lower premiums. The growth in electric mobility will continue to provide the basis for auto insurance. Companies can use wearables to monitor crucial health parameters and exercising habits. The creation of real-time dynamic rewards around it can change behaviour towards a healthier lifestyle. Awareness, longevity, rising costs and pandemic will only fuel this sector’s growth.
- Payments. Device to device contactless payment protocol is picking up and IoT and edge computing can create next-gen revolution in payments. Your EV could have an embedded wallet and pay for its parking and toll.
- Branch/ATM. IoT sensors and CCTV footage from branches/ATMs can be utilised in real-time to improve branch productivity as well as customer engagement, at the same time enhancing security. It could also help in other situations like low cash levels in ATMs and malfunctions. Sending live video streams for video analytics to the cloud can be expensive. By processing data within the device or on-premises, the Edge can help lower costs and reduce latency.
- Trading in Securities. Another area where response time matters is algorithmic trading. Edge computing will help to quickly process and analyse a large amount of data streaming real-time from multiple feeds and react appropriately.
- Trade Finance. Real-time tracking of goods may add a different dimension to the risk, pricing and transparency of supply chains.
Cloud vs Edge
The decision to use cloud or edge will depend on multiple considerations. At the same time, all the data from IoT devices need not go to the cloud for processing and choke network bandwidth. In fact, some of this data need not be stored forever (like video feeds etc). As a result, with the rise in the number of IoT devices and increasing financial access, edge computing will find its place in the sun and complement (and not compete) with cloud computing.
The views and opinions mentioned in the article are personal.
Anupam Verma is part of the Leadership team at ICICI Bank and his responsibilities have included leading the Bank’s strategy in South East Asia to play a significant role in capturing Investment, NRI remittance, and trade flows between SEA and India.
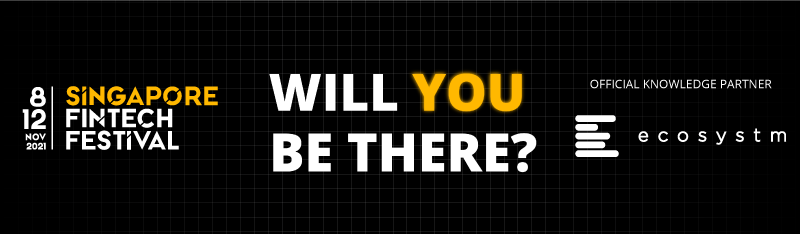
It is true that the Retail industry is being forced to evolve the experiences they deliver to their customers. However, if Retail organisations are only focused on creating digital experiences, they are not creating the differentiation that will be required to leap ahead of the competition.
It is time for Retail organisations to leverage data to empower multiple roles across the organisation to prepare for the different ways customers want to engage with their brands.
So what are the phases of customer engagement? How are companies such as Singapore Airlines and TikTok preparing for the future of Retail?
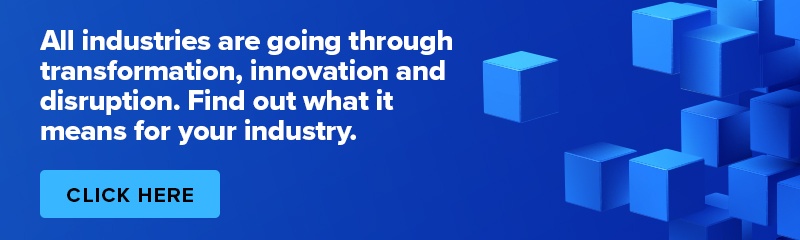
As we return to the office, there is a growing reliance on devices to tell us how safe and secure the environment is for our return. And in specific application areas, such as Healthcare and Manufacturing, IoT data is critical for decision-making. In some sectors such as Health and Wellness, IoT devices collect personally identifiable information (PII). IoT technology is so critical to our current infrastructures that the physical wellbeing of both individuals and organisations can be at risk.
Trust & Data
IoT are also vulnerable to breaches if not properly secured. And with a significant increase in cybersecurity events over the last year, the reliance on data from IoT is driving the need for better data integrity. Security features such as data integrity and device authentication can be accomplished through the use of digital certificates and these features need to be designed as part of the device prior to manufacturing. Because if you cannot trust either the IoT devices and their data, there is no point in collecting, running analytics, and executing decisions based on the information collected.
We discuss the role of embedding digital certificates into the IoT device at manufacture to enable better security and ongoing management of the device.
Securing IoT Data from the Edge
So much of what is happening on networks in terms of real-time data collection happens at the Edge. But because of the vast array of IoT devices connecting at the Edge, there has not been a way of baking trust into the manufacture of the devices. With a push to get the devices to market, many manufacturers historically have bypassed efforts on security. Devices have been added on the network at different times from different sources.
There is a need to verify the IoT devices and secure them, making sure to have an audit trail on what you are connecting to and communicating with.
So from a product design perspective, this leads us to several questions:
- How do we ensure the integrity of data from devices if we cannot authenticate them?
- How do we ensure that the operational systems being automated are controlled as intended?
- How do we authenticate the device on the network making the data request?
Using a Public Key Infrastructure (PKI) approach maintains assurance, integrity and confidentiality of data streams. PKI has become an important way to secure IoT device applications, and this needs to be built into the design of the device. Device authentication is also an important component, in addition to securing data streams. With good design and a PKI management that is up to the task you should be able to proceed with confidence in the data created at the Edge.
Johnson Controls/DigiCert have designed a new way of managing PKI certification for IoT devices through their partnership and integration of the DigiCert ONE™ PKI management platform and the Johnson Controls OpenBlue IoT device platform. Based on an advanced, container-based design, DigiCert ONE allows organisations to implement robust PKI deployment and management in any environment, roll out new services and manage users and devices across your organisation at any scale no matter the stage of their lifecycle. This creates an operational synergy within the Operational Technology (OT) and IoT spaces to ensure that hardware, software and communication remains trusted throughout the lifecycle.

Rationale on the Role of Certification in IoT Management
Digital certificates ensure the integrity of data and device communications through encryption and authentication, ensuring that transmitted data are genuine and have not been altered or tampered with. With government regulations worldwide mandating secure transit (and storage) of PII data, PKI can help ensure compliance with the regulations by securing the communication channel between the device and the gateway.
Connected IoT devices interact with each other through machine to machine (M2M) communication. Each of these billions of interactions will require authentication of device credentials for the endpoints to prove the device’s digital identity. In such scenarios, an identity management approach based on passwords or passcodes is not practical, and PKI digital certificates are by far the best option for IoT credential management today.
Creating lifecycle management for connected devices, including revocation of expired certificates, is another example where PKI can help to secure IoT devices. Having a robust management platform that enables device management, revocation and renewal of certificates is a critical component of a successful PKI. IoT devices will also need regular patches and upgrades to their firmware, with code signing being critical to ensure the integrity of the downloaded firmware – another example of the close linkage between the IoT world and the PKI world.
Summary
PKI certification benefits both people and processes. PKI enables identity assurance while digital certificates validate the identity of the connected device. Use of PKI for IoT is a necessary trend for sense of trust in the network and for quality control of device management.
Identifying the IoT device is critical in managing its lifespan and recognizing its legitimacy in the network. Building in the ability for PKI at the device’s manufacture is critical to enable the device for its lifetime. By recognizing a device, information on it can be maintained in an inventory and its lifecycle and replacement can be better managed. Once a certificate has been distributed and certified, having the control of PKI systems creates life-cycle management.
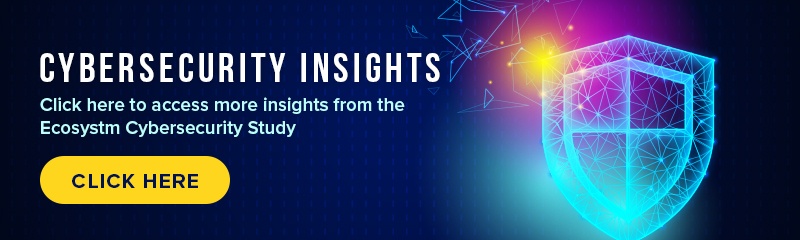
In this Insight, our guest author Anupam Verma talks about how the Global Capability Centres (GCCs) in India are poised to become Global Transformation Centres. “In the post-COVID world, industry boundaries are blurring, and business models are being transformed for the digital age. While traditional functions of GCCs will continue to be providing efficiencies, GCCs will be ‘Digital Transformation Centres’ for global businesses.”
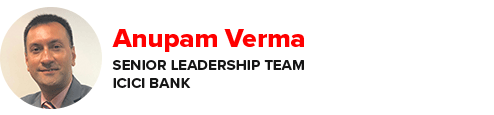
India has a lot to offer to the world of technology and transformation. Attracted by the talent pool, enabling policies, digital infrastructure, and competitive cost structure, MNCs have long embraced India as a preferred destination for Global Capability Centres (GCCs). It has been reported that India has more than 1,700 GCCs with an estimated global market share of over 50%.
GCCs employ around 1 million Indian professionals and has an immense impact on the economy, contributing an estimated USD 30 billion. US MNCs have the largest presence in the market and the dominating industries are BSFI, Engineering & Manufacturing, Tech & Consulting.
GCC capabilities have always been evolving
The journey began with MNCs setting up captives for cost optimisation & operational excellence. GCCs started handling operations (such as back-office and business support functions), IT support (such as app development and maintenance, remote IT infrastructure, and help desk) and customer service contact centres for the parent organisation.
In the second phase, MNCs started leveraging GCCs as centers of excellence (CoE). The focus then was product innovation, Engineering Design & R&D. BFSI and Professional Services firms started expanding the scope to cover research, underwriting, and consulting etc. Some global MNCs that have large GCCs in India are Apple, Microsoft, Google, Nissan, Ford, Qualcomm, Cisco, Wells Fargo, Bank of America, Barclays, Standard Chartered, and KPMG.
In the post-COVID world, industry boundaries are blurring, and business models are being transformed for the digital age. While traditional functions of GCCs will continue to be providing efficiencies, GCCs will be “Digital Transformation Centres” for global businesses.
The New Age GCC in the post-COVID world
On one hand, the pandemic broke through cultural barriers that had prevented remote operations and work. The world became remote everything! On the other hand, it accelerated digital adoption in organisations. Businesses are re-imagining customer experiences and fast-tracking digital transformation enabled by technology (Figure 1). High digital adoption and rising customer expectations will also be a big catalyst for change.
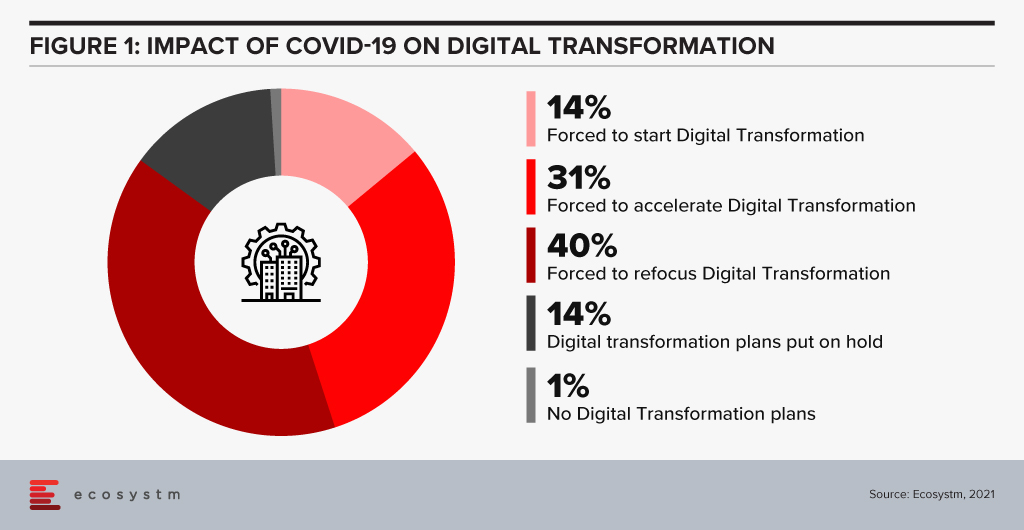
In last few years, India has seen a surge in talent pool in emerging technologies such as data analytics, experience design, AI/ML, robotic process automation, IoT, cloud, blockchain and cybersecurity. GCCs in India will leverage this talent pool and play a pivotal role in enabling digital transformation at a global scale. GCCs will have direct and significant impacts on global business performance and top line growth creating long-term stakeholder value – and not be only about cost optimisation.
GCCs in India will also play an important role in digitisation and automation of existing processes, risk management and fraud prevention using data analytics and managing new risks like cybersecurity.
More and more MNCs in traditional businesses will add GCCs in India over the next decade and the existing 1,700 plus GCCs will grow in scale and scope focussing on innovation. Shift of supply chains to India will also be supported by Engineering R & D Centres. GCCs passed the pandemic test with flying colours when an exceptionally large workforce transitioned to the Work from Home model. In a matter of weeks, the resilience, continuity, and efficiency of GCCs returned to pre-pandemic levels with a distributed and remote workforce.
A Final Take
Having said that, I believe the growth spurt in GCCs in India will come from new-age businesses. Consumer-facing platforms (eCommerce marketplaces, Healthtechs, Edtechs, and Fintechs) are creating digital native businesses. As of June 2021, there are more than 700 unicorns trying to solve different problems using technology and data. Currently, very few unicorns have GCCs in India (notable names being Uber, Grab, Gojek). However, this segment will be one of the biggest growth drivers.
Currently, only 10% of the GCCs in India are from Asia Pacific organisations. Some of the prominent names being Hitachi, Rakuten, Panasonic, Samsung, LG, and Foxconn. Asian MNCs have an opportunity to move fast and stay relevant. This segment is also expected to grow disproportionately.
New age GCCs in India have the potential to be the crown jewel for global MNCs. For India, this has a huge potential for job creation and development of Smart City ecosystems. In this decade, growth of GCCs will be one of the core pillars of India’s journey to a USD 5 trillion economy.
The views and opinions mentioned in the article are personal.
Anupam Verma is part of the Senior Leadership team at ICICI Bank and his responsibilities have included leading the Bank’s strategy in South East Asia to play a significant role in capturing Investment, NRI remittance, and trade flows between SEA and India.
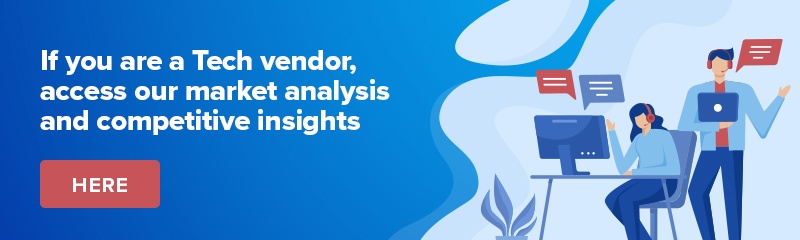
Organisations have found that it is not always desirable to send data to the cloud due to concerns about latency, connectivity, energy, privacy and security. So why not create learning processes at the Edge?
What challenges does IoT bring?
Sensors are now generating such an increasing volume of data that it is not practical that all of it be sent to the cloud for processing. From a data privacy perspective, some sensor data is sensitive and sending data and images to the cloud will be subject to privacy and security constraints.
Regardless of the speed of communications, there will always be a demand for more data from more sensors – along with more security checks and higher levels of encryption – causing the potential for communication bottlenecks.
As the network hardware itself consumes power, sending a constant stream of data to the cloud can be taxing for sensor devices. The lag caused by the roundtrip to the cloud can be prohibitive in applications that require real-time response inputs.
Machine learning (ML) at the Edge should be prioritised to leverage that constant flow of data and address the requirement for real-time responses based on that data. This should be aided by both new types of ML algorithms and by visual processing units (VPUs) being added to the network.
By leveraging ML on Edge networks in production facilities, for example, companies can look out for potential warning signs and do scheduled maintenance to avoid any nasty surprises. Remember many sensors are linked intrinsically to public safety concerns such as water processing, supply of gas or oil, and public transportation such as metros or trains.
Ecosystm research shows that deploying IoT has its set of challenges (Figure 1) – many of these challenges can be mitigated by processing data at the Edge.
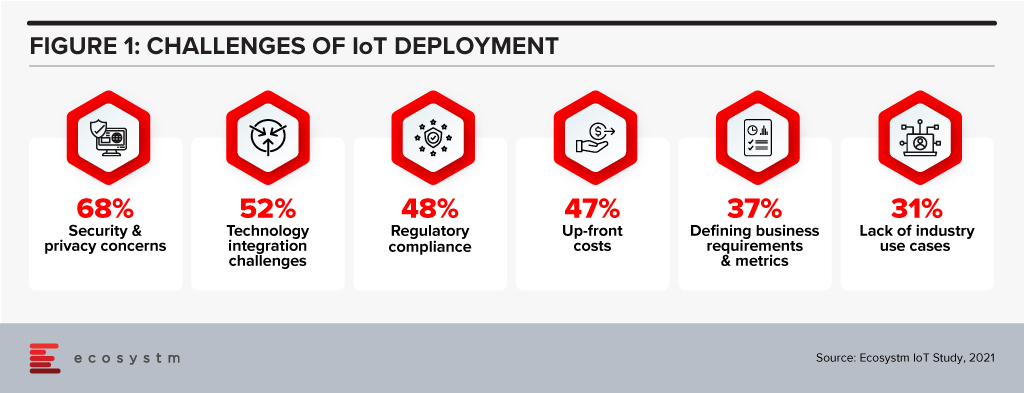
Predictive analytics is a fundamental value proposition for IoT, where responding faster to issues or taking action before issues occur, is key to a high return on investment. So, using edge computing for machine learning located within or close to the point of data gathering can in some cases be a more practical or socially beneficial approach.
In IoT the role of an edge computer is to pre-process data and act before the data is passed on to the main server. This allows a faster, low latency response and minimal traffic between the cloud server processing and the Edge. However, a better understanding of the benefits of edge computing is required if it has to be beneficial for a number of outcomes.
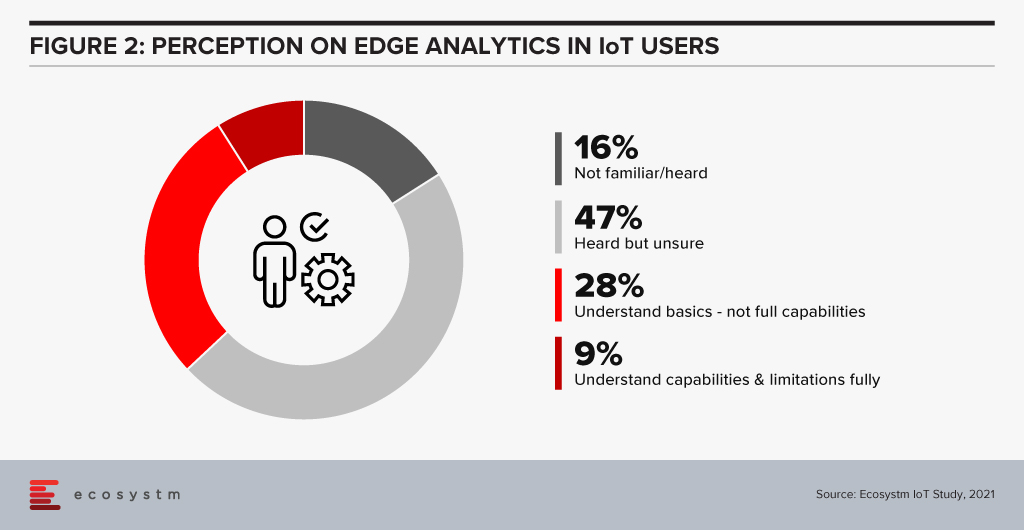

If we can get machine learning happening in the field, at the Edge, then we reduce the time lag and also create an extra trusted layer in unmanned production or automated utilities situations. This can create more trusted environments in terms of possible threats to public services.
What kind of examples of machine learning in the field can we see?
Healthcare
Health systems can improve hospital patient flow through machine learning (ML) at the Edge. ML offers predictive models to assist decision-makers with complex hospital patient flow information based on near real-time data.
For example, an academic medical centre created an ML pipeline that leveraged all its data – patient administration, EHR and clinical and claims data – to create learnings that could predict length of stay, emergency department (ED) arrival models, ED admissions, aggregate discharges, and total bed census. These predictive models proved effective as the medical centre reduced patient wait times and staff overtime and was able to demonstrate improved patient outcomes. And for a medical centre that use sensors to monitor patients and gather requests for medicine or assistance, Edge processing means keeping private healthcare data in-house rather than sending it off to cloud servers.
Retail
A retail store could use numerous cameras for self-checkout and inventory management and to monitor foot traffic. Such specific interaction details could slow down a network and can be replaced by an on-site Edge server with lower latency and a lower total cost. This is useful for standalone grocery pop-up sites such as in Sweden and Germany.
In Retail, k-nearest neighbours is often used in ML for abnormal activity analysis – this learning algorithm can also be used for visual pattern recognition used as part of retailers’ loss prevention tactics.
Summary
Working with the data locally on the Edge, creates reduced latency, reduced cloud usage and costs, independence from a network connection, more secure data, and increased data privacy.
Cloud and Edge computing that uses machine learning can together provide the best of both worlds: decentralised local storage, processing and reaction, and then uploading to the cloud, enabling additional insights, data backups (redundancy), and remote access.
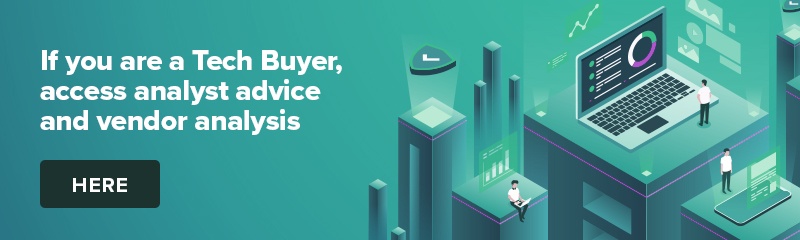
Last week I wrote about the need to remove hype from reality when it comes to AI. But what will ensure that your AI projects succeed?
It is quite obvious that success is determined by human aspects rather than technological factors. We have identified four key organisational actions that enable successful AI implementation at scale (Figure 1).
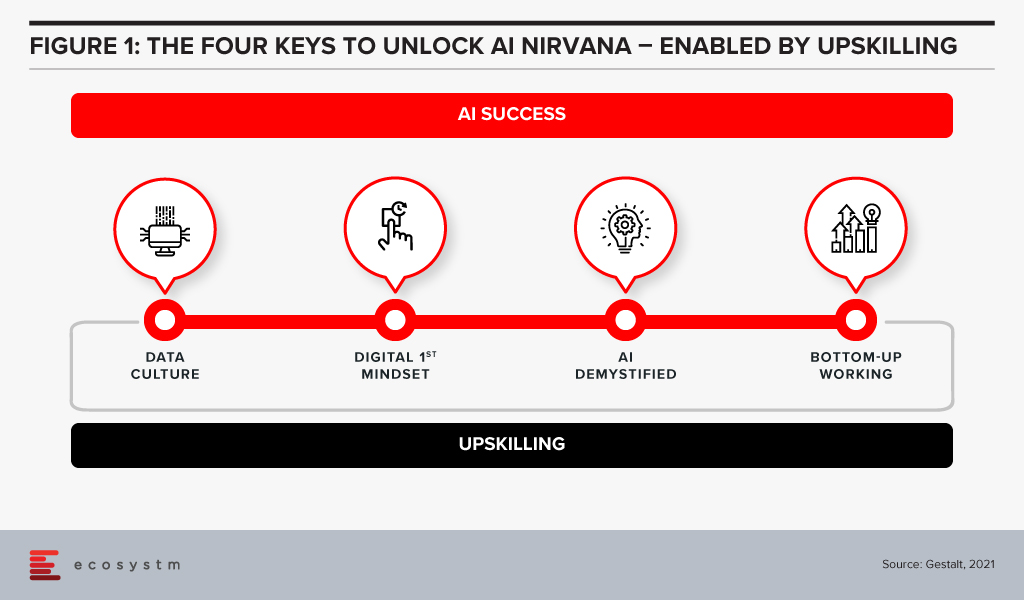
#1 Establish a Data Culture
The traditional focus for companies has been on ensuring access to good, clean data sets and the proper use of that data. Ecosystm research shows that only 28% of organisations focused on customer service, also focus on creating a data-driven organisational culture. But our experience has shown that culture is more critical than having the data. Does the organisation have a culture of using data to drive decisions? Does every level of the organisation understand and use data insights to do their day-to-day jobs? Is decision-making data-driven and decentralised, needing to be escalated only when there is ambiguity or need for strategic clarity? Do business teams push for new data sources when they are not able to get the insights they need?
Without this kind of culture, it may be possible to implement individual pieces of automation in a specific area or process, applying brute force to see it through. In order to transform the business and truly extract the power of AI, we advise organisations to build a culture of data-driven decision-making first. That organisational mindset, will make you capable implementing AI at scale. Focusing on changing the organisational culture will deliver greater returns than trying to implement piecemeal AI projects – even in the short to mid-term.
#2 Ingrain a Digital-First Mindset
Assuming a firm has passed the data culture hurdle, it needs to consider whether it has adopted a digital-first mindset. AI is one of many technologies that impact businesses, along with AR/VR, IoT, 5G, cloud and Blockchain to name a few. Today’s environment requires firms to be capable of utilising a variety of these technologies – often together – and possessing a workforce capable of using these digital tools.
A workforce with the digital-first mindset looks for a digital solution to problems wherever appropriate. They have a good understanding of digital technologies relevant to their space and understand key digital methodologies – such as Customer 360 to deliver a truly superior customer experience or Agile methodologies to successfully manage AI at scale.
AI needs business managers at the operational levels to work with IT or AI tech teams to pinpoint processes that are right for AI. They need to make an estimation based on historical data of what specific problems require an AI solution. This is enabled by the digital-first mindset.
#3 Demystify AI
The next step is to get business leaders, functional leaders, and business operational teams – not just those who work with AI – to acquire a basic understanding of AI.
They do not need to learn the intricacies of programming or how to create neural networks or anything nearly as technical in nature. However, all levels from the leadership down should have a solid understanding of what AI can do, the basics of how it works, how the process of training data results in improved outcomes and so on. They need to understand the continuous learning nature of AI solutions, getting better over time. While AI tools may recommend an answer, human insight is often needed to make a correct decision off this recommendation.

#4 Drive Implementation Bottom-Up
AI projects need alignment, objectives, strategy – and leadership and executive buy-in. But a very important aspect of an AI-driven organisation that is able to build scalable AI, is letting projects run bottom up.
As an example, a reputed Life Sciences company embarked on a multi-year AI project to improve productivity. They wanted to use NLP, Discovery, Cognitive Assist and ML to augment clinical proficiency of doctors and expected significant benefits in drug discovery and clinical trials by leveraging the immense dataset that was built over the last 20 years.
The company ran this like any other transformation project, with a central program management team taking the lead with the help of an AI Centre of Competency. These two teams developed a compelling business case, and identified initial pilots aligned with the long-term objectives of the program. However, after 18 months, they had very few tangible outcomes. Everyone including doctors, research scientists, technicians, and administrators, who participated in the program had their own interpretation of what AI was not able to do.
Discussion revealed that the doctors and researchers felt that they were training AI to replace themselves. Seeing a tool trying to mimic the same access and understanding of numerous documents baffled them at best. They were not ready to work with AI programs step-by-step to help AI tools learn and discover new insights.
At this point, we suggested approaching the project bottom-up – wherein the participating teams would decide specific projects to take up. This developed a culture where teams collaborated as well as competed with each other, to find new ways to use AI. Employees were shown a roadmap of how their jobs would be enhanced by offloading routine decisions to AI. They were shown that AI tools augment the employees’ cognitive capabilities and made them more effective.
The team working on critical trials found these tools extremely useful and were able to collaborate with other organisations specialising in similar trials. They created the metadata and used ML algorithms to discover new insights. Working bottom-up led to a very successful AI deployment.
We have seen time and again that while leadership may set the strategy and objectives, it is best to let the teams work bottom-up to come up with the projects to implement.
#5 Invest in Upskilling
The four “keys” are important to build an AI-powered, future-proof enterprise. They are all human related – and when they come together to work as a winning formula is when organisations invest in upskilling. Upskilling is the common glue and each factor requires specific kinds of upskilling (Figure 2).
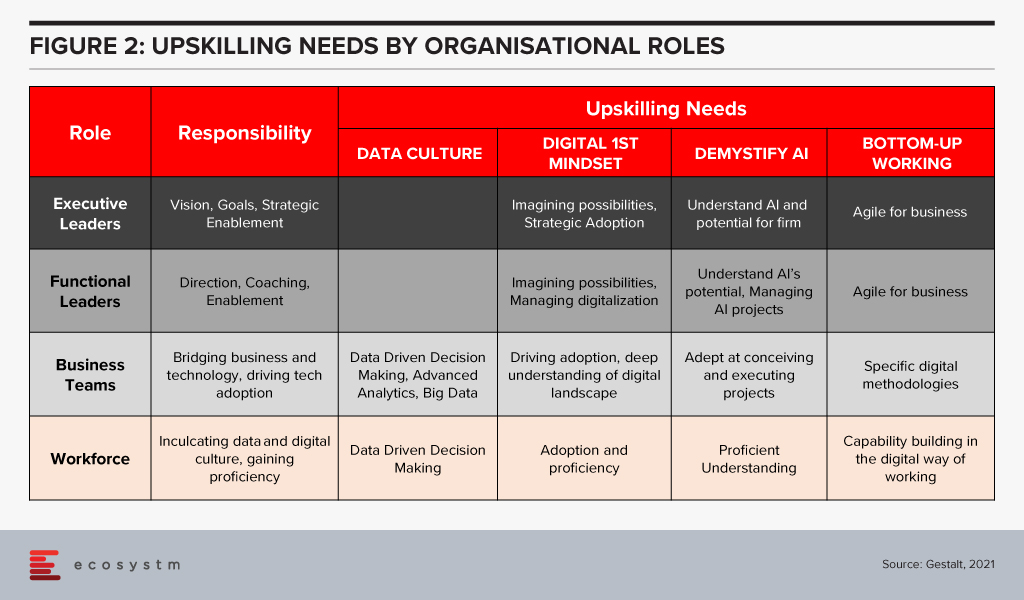
Upskilling needs vary by organisational level and the key being addressed. The bottom line is that upskilling is a universal requirement for driving AI at scale, successfully. And many organisations are realising it fast – Bosch and DBS Bank are some of the notable examples.
How much is your organisation invested in upskilling for AI implementation at scale? Share your stories in the comment box below.
Written with contributions from Ravi Pattamatta and Ratnesh Prasad
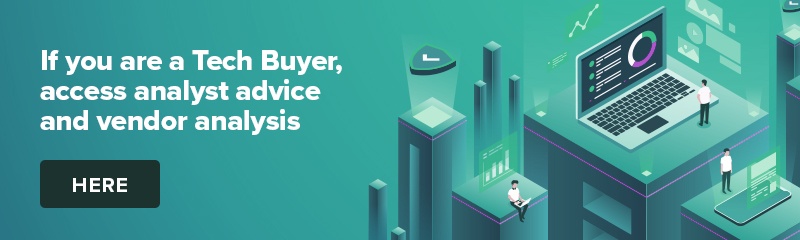